AI Resources
UTRGV Cradle Cluster
All resource projects developed under STARTER will have access to the UTRGV Cradle Cluster. Our Cradle Cluster is designed to handle complex computations, analyze large datasets, and run simulations at lightning speed.
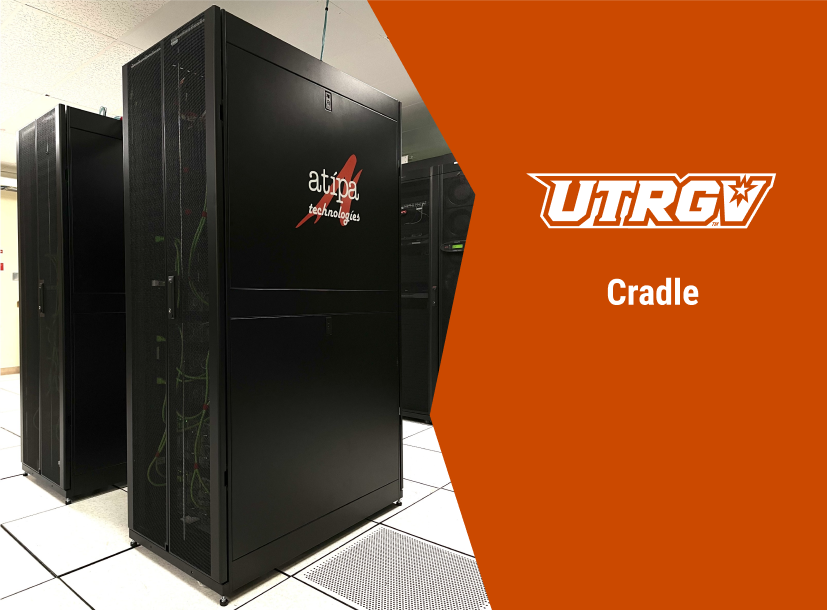
TACC Lonestar6 Cluster
UTRGV faculty and students working under STARTER can also request time allocations (at no monetary cost) on the Lonestar6 cluster at the Texas Advanced Computing Center (TACC).
Access-Controlled Workstations
Access-controlled computer labs in both Brownsville and Edinburg campuses will be made available to students. Each workstation will have a GPU and will have access to the UTRGV Cradle Cluster and be able to smoothly migrate data. All GPU workstations will be managed by UTRGV IT staff.
Workshops
STARTER will organize 2 workshops from 2024-2025, one in the summer of 2024 (STARTER-AI Workshop 2024) and another in the spring of 2025 (STARTER-AI Workshop 2025) thanks to the support of NSF, NIH, and NVIDIA. These workshops will be held across UTRGV's Edinburg and Brownsville campuses and will provide live audio-visual connections. Workshops will be advertised here on our homepage under Events. STARTER workshops will include the following.
Tutorials & Hands-On Training
Our faculty personnel will deliver tutorials and hands-on training sessions on various aspects of AI and machine learning from the micro-credential format courses to all attending participants.
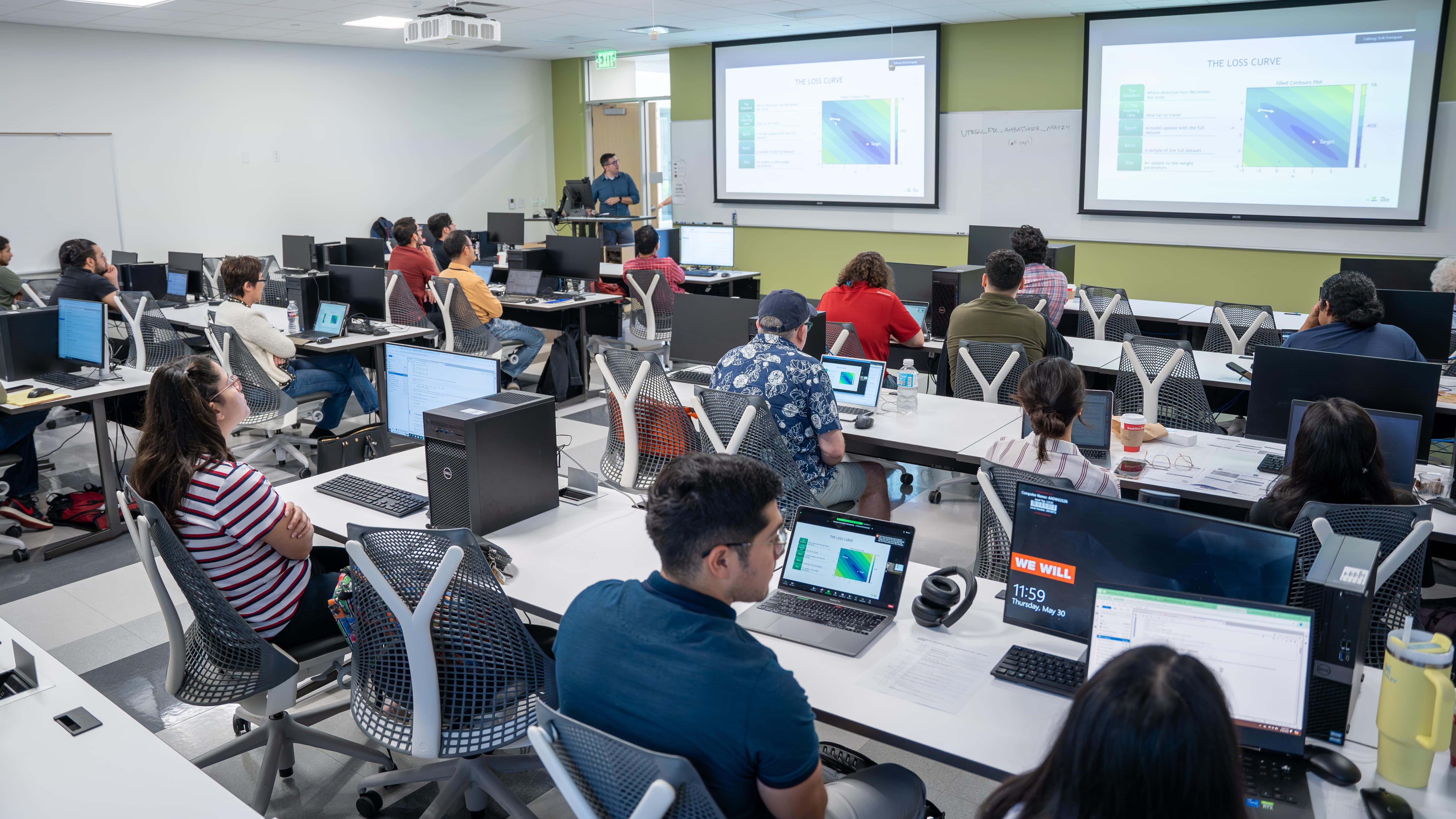
"Bring Your Own Problems"
"Bring your own problems" sessions will be organized and advertized before the workshop to inform and attract researchers interested in using AI and machine learning. These sessions aim to identify startup projects within the community and provide them with the adequate support.
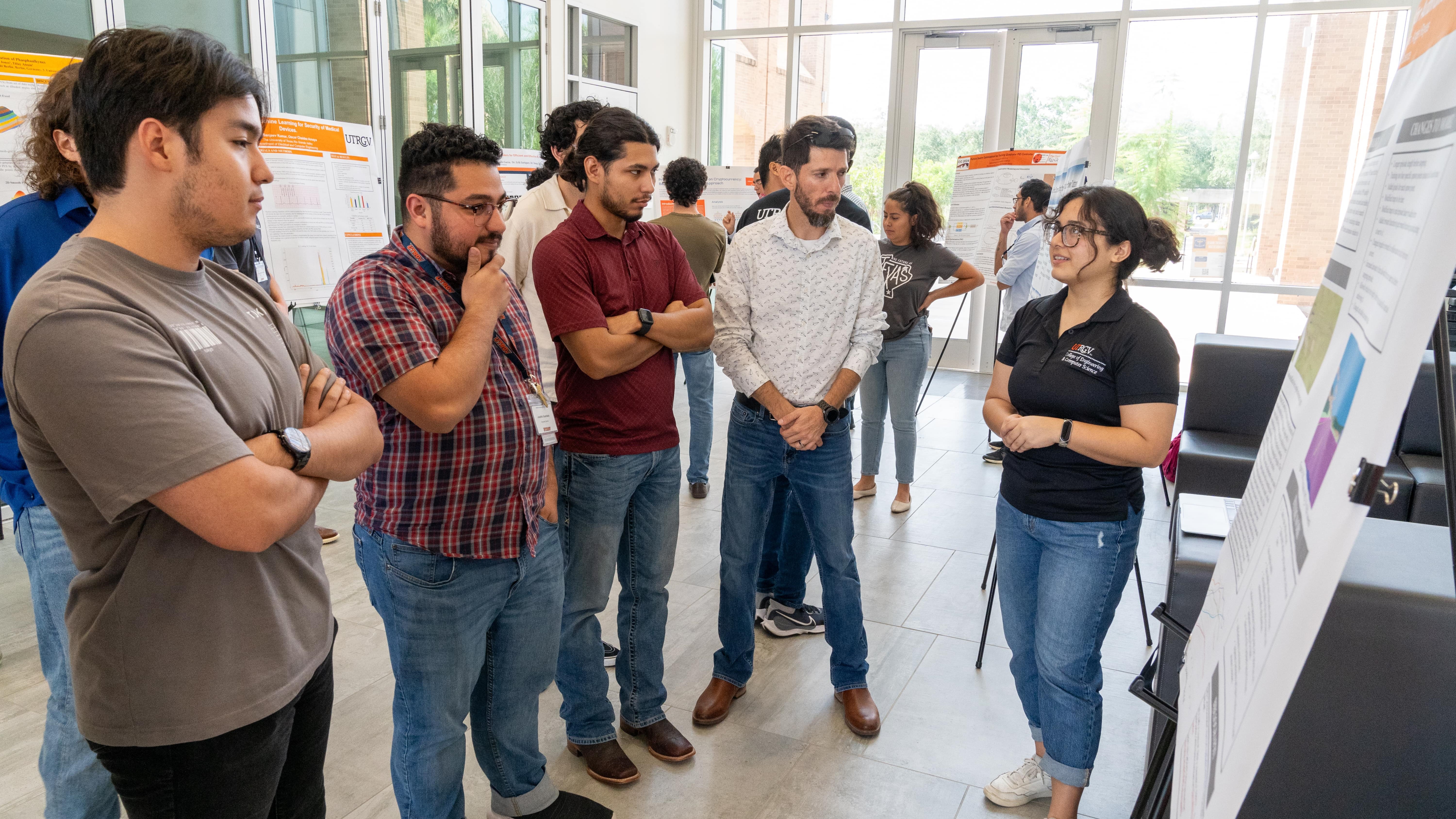
External Speakers
External speakers will be invited to talk about AI technologies and their impacts in diverse fields, including ethical aspects of AI technology. Besides gaining insights into real-world applications of AI, interactions with external speakers may open collaboration opportunities for UTRGV faculty and students.